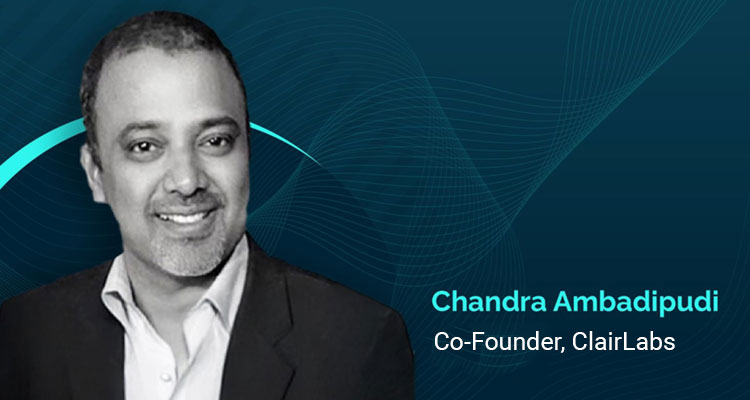
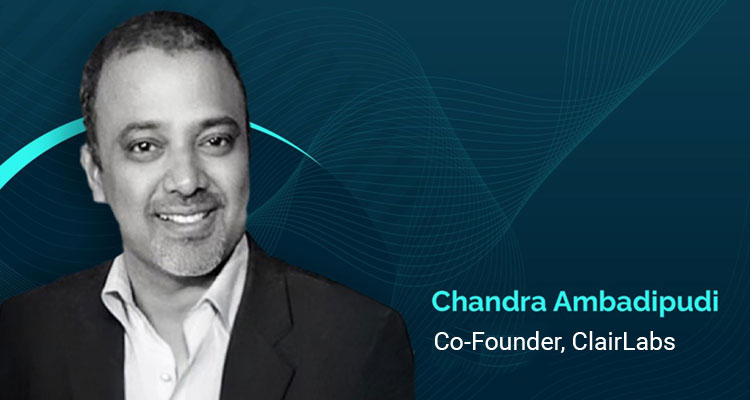
The role of technology in health and life care has evolved from a single-point to a multidimensional, holistic approach with the advent of AI. However, despite its rapid evolution, the health landscape has a long way to go, with over 4.5 billion people lacking access to essential healthcare services. The growing shortage of skilled healthcare workers, skyrocketing bills, supply chain disruptions, and regulatory hurdles are a cry for help — for innovation, demanding a breakthrough. And AI-powered genomics is answering that call, urging healthcare and life sciences leaders to recalibrate how diseases are diagnosed, treated, and managed by tailoring interventions to individual patients’ genetic, environmental, and lifestyle factors.
Health and life sciences enterprise leaders are laser-focused on precise disease prediction, tailored treatments, and advanced genetic research. Notably, by 2025, AI-driven genomic analysis is expected to enhance precision medicine (1), particularly in cancer treatment, by identifying complex genetic variants associated with diseases. Here are some key innovations in AI-powered genomics:
AI-driven genetic analysis
Today, organisations are leveraging AI-led algorithms to analyse vast genomic datasets from diverse patient populations and suggest suitable treatment modalities and personalised therapies. For instance, by combining Machine Learning (ML) and deep learning algorithms, AI can help users analyse genomic data and identify biomarkers to elucidate intricate relationships between genetic factors and drug responses. Such breakthroughs underscore the increasing role of AI in informing treatment decisions, mitigating adverse reactions, and refining drug dosages (2) in clinical settings.
Personalised medicine
When enhanced focus is given to diagnostic accuracy, prognostic assessments, and therapeutic planning, it can empower health centres to enable more precise patient stratification and improved clinical outcomes. This advancement is particularly evident in pharmacogenomics, as it paves the way for the development of self-healing medicines. For instance, DeepMind’s AI system accurately predicts 3D protein structures based on their amino acid sequences and folding, unravelling insights into how drugs interact with their targets. Besides opening new avenues for targeted therapies(3), this has also accelerated research in various biomedical fields.
Genomic insights for Precision medicine
Researchers globally have been leveraging AI-powered tools to integrate genomic sequencing data with clinical records to obtain actionable insights. For instance, AI augmentation in cancer screening workflows is helping health centres explore alternative forms of investigation. Liquid biopsy (4), which involves studying tumour cells across bodily fluids—including blood, pleural, peritoneal, or cerebrospinal fluid, nipple aspirate, or urine—is becoming increasingly popular. Besides being a non-invasive method, it also provides doctors with a comprehensive picture of genetic variations in tumours, allowing them to take multiple samples over time to monitor disease evolution.
Machine learning in Genetic research
To expedite turnaround cycles for drug discovery and disease prevention, organisations have intensified their experimentation with AI to predict gene functions and detect mutations. For instance, in the largest study of its kind, researchers from Genentech, Roche, and Stanford University analysed data from 78,000 patients with cancer across 20 different types. The team developed an ML tool and employed computational analysis to identify 95 genes associated with breast, ovarian, skin, and gastrointestinal cancers. They also identified nearly 800 genetic variations that could directly impact survival rates.
By 2025, AI-driven genomics is set to redefine personalised health and life care, though enterprises must overcome significant hurdles such as data inaccessibility, silos, inaccuracy, and inconsistency. They must also resolve system integration and data privacy issues, as well as concerns regarding AI model explainability and transparency, user acceptance, and training, in addition to the higher cost of infrastructure.
While AI-augmented health modalities appear promising, health and life sciences organisations must also consider regulatory, legal, and ethical implications. AI has the potential to transform disease diagnosis, treatment, and management into a more precise, individualised process. With the right strategy, technology partners, investment pipelines, and sustainability-centric workflows, the future of holistic preventive healthcare would no longer be a distant dream.
References
- https://www.nature.com/articles/s41746-025-01471
- https://www.sciencedirect.com/science/article/pii/S2949866X2400087X
- https://jpionline.org/10.5530/ijpi.20250100#R4
- https://www.nature.com/articles/s44276-025-00135-4